报告人:许杏柏副教授
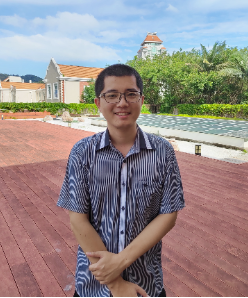
工作单位:厦门大学王亚南经济研究院和经济学院
报告题目:Transfer Learning for Spatial
Autoregressive Models
报告时间:2024年6月19日(周三)15:00
报告地点:文理楼290
腾讯会议号:666821523
内容摘要:The spatial autoregressive (SAR) model has been widely applied in various empirical economic studies to characterize the spatial dependence among subjects. However, the precision of estimating the SAR model diminishes when the sample size of the target data is limited. In this paper, we propose a new transfer learning framework for the SAR model to borrow the information from similar source data to improve both estimation and prediction. When the informative source data sets are known, we introduce a two-stage algorithm, including a transferring stage and a debiasing stage, to estimate the unknown parameters and also establish the theoretical convergence rates for the resulting estimators. If we do not know which sources to transfer, a transferable source detection algorithm is proposed to detect informative sources data based on spatial residual bootstrap to retain the necessary spatial dependence. Its detection consistency is also derived. Simulation studies demonstrate that using informative source data, our transfer learning algorithm significantly enhances the performance of the classical two-stage least squares estimator. In the empirical application, we apply our method to the election prediction in swing states in the 2020 U.S. presidential election, utilizing polling data from the 2016 U.S. presidential election along with other demographic and geographical data. The empirical results show that our method outperforms traditional estimation methods.
个人简介:许杏柏,厦门大学王亚南经济研究院和经济学院长聘副教授,博士生导师。2008年获清华大学数学学士学位,2010年获北京大学经济学硕士学位,2016年从俄亥俄州立大学获得经济学博士学位。主持国家自然科学青年基金1项,现在主持国家自然科学基金面上项目1项、国家自然科学基金重点项目子课题1项。主要研究领域为空间计量经济学和网络计量经济学。多份研究成果发表在Journal of Econometrics(经济学顶刊), Econometric Theory, Regional Science and Urban Economics等经济学、统计学国际权威学术期刊上。