报告人:Nikhil R. Pal 院士
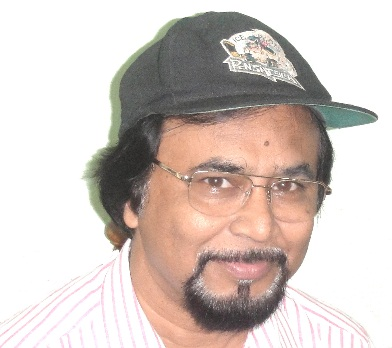
工作单位:印度统计研究所
报告题目:C-均值聚类算法的可视化核变体表达
报告时间:2022年7月30日(周六)上午10:30-11:30
报告链接:
https://teams.microsoft.com/l/meetup-join/19%3aB4gmRcUATAMA2iJqi-xXvtfPFfTbxVJPxSW_pcAPBao1%40thread.tacv2/1638719716825?context=%7b%22Tid%22%3a%2222804ebb-30d5-47df-942f-f3a3722f0225%22%2c%22Oid%22%3a%2216a60c03-ad7a-4b85-a403-8ebd947e010c%22%7d
内容摘要:
目前已经提出了C-均值(硬和模糊)聚类算法的不同核变体。针对n维对象数据X的核聚类,首先,提出一个基本问题:是否可以在核空间中对任何给定的对象数据进行聚类?答案是否定的!这是因为当尝试在变换空间进行聚类时,要确保其是否有助于得到同原始数据X中一致的聚类结果;否则,可能会产生完全不相关的聚类,从而导致核聚类无效。这一问题,既不依赖于聚类算法的选择,也不依赖于所使用的特定变换(核函数)。除了2维或3维的数据,我们几乎没有任何“简单”的方法来回答这个问题。对于2维或3维的数据,由于数据的可视化,核聚类并没有真正带来什么好处。因此,除非能够解决一些基本问题,否则核聚类似乎没有任何优势。本报告将介绍使用具有视觉评估以及标准化互信息(NMI)、调整兰德指数(ARI)和聚类不稳定性的合成数据集和真实数据集来阐述和验证以上观点,并使用Sammon非线性投影方法来获得核空间中数据的粗略视觉表达。最后,将讨论核函数和算法参数如何相互作用以及如何选择合适的核函数参数的问题。
个人简介:
Nikhil R. Pal,印度统计研究所电子与通信学部教授、IEEE Fellow, 印度科学院院士、印度工程院院士、发展中国家科学院院士,现担任印度统计研究所人工智能与机器学习中心主任。Nikhil R. Pal 院士研究方向包括脑科学、计算智能、机器学习和数据挖掘。2005年1月至2010年12月期间,担任IEEE Transactions on Fuzzy Systems的主编。他曾/一直在多个期刊的编辑/顾问委员会/指导委员会任职,包括International Journal of Approximate Reasoning,Applied Soft Computing,International Journal of Neural Systems, Fuzzy Sets and Systems, IEEE Transactions on Fuzzy Systems和IEEE Transactions on Cybernetics。Nikhil R. Pal院士是2015年IEEE计算智能学会(CIS)模糊系统先锋奖和2021年IEEE CIS卓越服务奖的获得者。他在计算智能领域的不同主要国际会议上做了多个大会主题报告。曾担任多个会议的总主席、项目主席和联合项目主席。曾是IEEE CIS的杰出讲师(2010-2012、2016-2018、2022-2024),并且是IEEE CIS管理委员会委员(2010-2012)。Nikhil R. Pal 院士曾担任 IEEE CIS 出版副主席(2013-2016)和 IEEE CIS主席(2018-2019)。
(www.isical.ac.in/~nikhil)
【编辑:王健】
英文版:
Speaker: Academician Nikhil R Pal
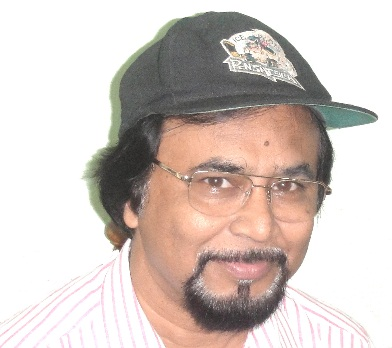
Title: What and when can we gain from the kernel versions of c-means algorithm?
Time: 10:30 am, July 30, 2022 (Saturday)
Link: https://teams.microsoft.com/l/meetup-join/19%3aB4gmRcUATAMA2iJqi-xXvtfPFfTbxVJPxSW_pcAPBao1%40thread.tacv2/1638719716825?context=%7b%22Tid%22%3a%2222804ebb-30d5-47df-942f-f3a3722f0225%22%2c%22Oid%22%3a%2216a60c03-ad7a-4b85-a403-8ebd947e010c%22%7d
Abstract:
Different kernelized versions of c-means (hard and fuzzy) clustering algorithms have been proposed. Here we focus on kernel-clustering of only n-dimensional object data. First, we raise a basic question: should we really cluster any given object data in the kernel space? Our answer answer is NO! We shall provide our line of arguments. We shall establish that when we try to cluster in a transformed space, we must know if it could help us to find the clusters present in the original data X. To get any benefit from kernel clustering (or clustering in any other transformed space) we need to answer this question first; otherwise, we may find completely irrelevant clusters without knowing it and thereby making kernel clustering useless. This issue is a philosophical one and is neither dependent on the choice of clustering algorithm nor on the particular transformation (kernel function) used. Except for 2D/3D data, we do not have any “easy” way to answer the question and for 2D/3D data since we can look at the data we really do not really get any benefit from kernel clustering. So it appears that there is no benefit from kernel clustering unless we can answer some basic questions! We demonstrate and justify our claims using both synthetic and real data sets with visual assessment as well as with Normalized Mutual Information (NMI), Adjusted Rand Index (ARI) and cluster instability. We propose to use Sammon's nonlinear projection method to get a crude visual representation of the data in the kernel space. We discuss the issue of how to choose appropriate parameters of the kernel function, but we could not provide a solution to this problem. Finally, we discuss how the kernel parameters and the algorithmic parameters interact.
Personal Introduction:
Nikhil R. Pal is a Professor in the Electronics and Communication Sciences Unit and is the Head of the Center for Artificial Intelligence and Machine Learning of the Indian Statistical Institute. His current research interest includes brain science, computational intelligence, machine learning and data mining. He was the Editor-in-Chief of the IEEE Transactions on Fuzzy Systems for the period January 2005-December 2010. He has served/been serving on the editorial /advisory board/ steering committee of several journals including the International Journal of Approximate Reasoning, Applied Soft Computing, International Journal of Neural Systems, Fuzzy Sets and Systems, IEEE Transactions on Fuzzy Systems and the IEEE Transactions on Cybernetics. He is a recipient of the 2015 IEEE Computational Intelligence Society (CIS) Fuzzy Systems Pioneer Award and 2021 IEEE CIS Meritorious Service Award. He has given many plenary/keynote speeches in different premier international conferences in the area of computational intelligence. He has served as the General Chair, Program Chair, and co-Program chair of several conferences. He has been a Distinguished Lecturer of the IEEE CIS (2010-2012, 2016-2018, 2022-2024) and was a member of the Administrative Committee of the IEEE CIS (2010-2012). He has served as the Vice-President for Publications of the IEEE CIS (2013-2016) and the President of the IEEE CIS (2018-2019). He is a Fellow of the West Bengal Academy of Science and Technology, Institution of Electronics and Tele Communication Engineers, National Academy of Sciences, India, Indian National Academy of Engineering, Indian National Science Academy, International Fuzzy Systems Association (IFSA), The World Academy of Sciences, and a Fellow of the IEEE, USA. (www.isical.ac.in/~nikhil)
[Editor: Jian Wang]